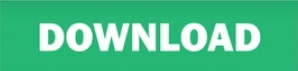
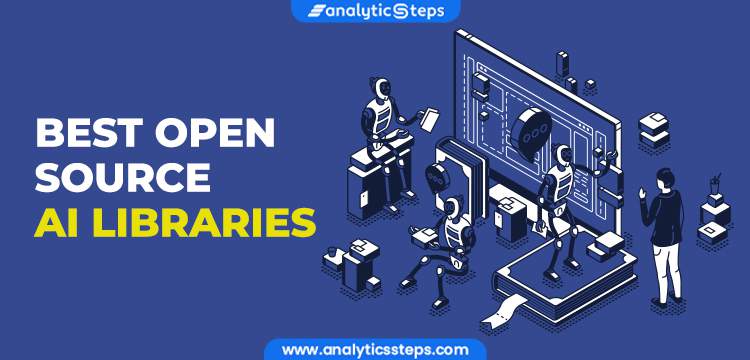
Acquiring such a dataset can be challenging, so researchers usually turn to transfer learning: fine-tuning models that have been pretrained on a large publicly-available dataset of audio only. Training a deep-learning speech-recognition model using only supervised learning would require a large dataset containing audio data with corresponding accurate or gold standard transcripts.
#Open source ai transcription code
We are open-sourcing models and inference code to serve as a foundation for building useful applications and for further research on robust speech processing.We hope Whisper’s high accuracy and ease of use will allow developers to add voice interfaces to a much wider set of applications. In zero-shot evaluations on a set of speech recognition datasets, Whisper made on average 55% fewer errors than Wav2Vec, a baseline model. Unlike most state-of-the-art ASR models, Whisper is not fine-tuned on any benchmark dataset instead, it is trained using "weak" supervision on a large-scale, noisy dataset of speech audio and paired transcription text collected from the internet. Whisper uses an encoder-decoder Transformer architecture and processes audio in 30-second chunks. Whisper was trained on 680,000 hours of audio data collected from the web and shows robust zero-shot performance on a wide range of automated speech recognition (ASR) tasks. OpenAI recently released Whisper, a 1.6 billion parameter AI model that can transcribe and translate speech audio from 97 different languages.
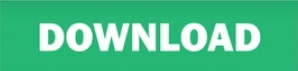